Synergistic Activities
- Academic Background: Dr. Bo-Wen Shen is an Associate Professor in the Department of Mathematics and Statistics at San Diego State University (SDSU). He earned his Ph.D. from North Carolina State University in 1998. With over 25 years of experience, Dr. Shen has developed expertise in mesoscale and global modeling, high-performance computing, numerical weather prediction, nonlinear dynamics, and artificial intelligence (AI) and deep learning (DL). He has edited a book celebrating the 50th anniversary of the butterfly effect, published more than 60 peer-reviewed journal articles, and completed over 50 technical or conference reports. Additionally, he has served on the editorial boards of five international journals.
- NASA Contributions: Dr. Shen joined the modeling team at NASA's Goddard Space Flight Center (GSFC) in 1999, where he contributed to the development of a unified weather and climate model. Early in his career at GSFC, he and his colleagues successfully used a global mesoscale model for real-time hurricane prediction, earning recognition from the American Geophysical Union, Science, and other media outlets. From 2009 to 2015, Dr. Shen was the principal investigator for NASA's CAMVis (Coupled Advanced Global Modeling and Concurrent Visualization Systems) projects, where he led efforts to improve the computational efficiency of the Goddard Multiscale Modeling Framework (MMF) and introduced three-level parallelism to the Ensemble Empirical Mode Decomposition (EMD).
- Contributions at SDSU: In 2011, Dr. Shen shifted his focus to nonlinear dynamics and chaos theory to better understand the predictability of high-resolution global model simulations. He joined the faculty at SDSU in 2014, where he has since published significant works, including the development of a generalized Lorenz model. He and his co-authors proposed referring to the two-week predictability limit as the Predictability Limit Hypothesis, drawing an analogy to Moore's law, and presented a revised perspective on "the dual nature of chaos and order," challenging the traditional view that "weather is chaotic."
- Recent AI and ML Work: Since the early 2010s, beginning with his second AIST computing project that focused on the development of the parallel ensemble empirical mode decomposition (PEMMD) for multiscale analysis, Dr. Shen has been exploring machine learning (ML) and mentoring students in applying ML techniques, such as Kernel Principal Component Analysis and recurrence plots, to analyze chaotic solutions. Since his sabbatical leave in 2022, he has advanced in developing deep learning (DL) models based on transformers and has published research in this area to examine the role of upscaling and downscaling in assessing predictability in the real-world global weather and climate models.
- Research Awards: His early papers and results utilizing the high-resolution global model were featured by Science Magazine and the American Geophysical Union in 2006 and were recognized as pioneering work by Roger Pielke Sr. He received ten outstanding performance awards from NASA/GSFC and the University of Maryland, College Park, between 2001 and 2010. (https://bwshen.sdsu.edu/shen_honors_and_awards.html)
- Feature Articles: His recent papers have been selected as feature articles (Shen et al. 2024; Shen 2023), editor’s choice articles (Shen et al. 2022), or annually featured articles (Shen 2019; Faghih-Naini and B.-W. Shen 2018). (https://bwshen.sdsu.edu/shen_research_new_and_highlights.html)
(Link)
|
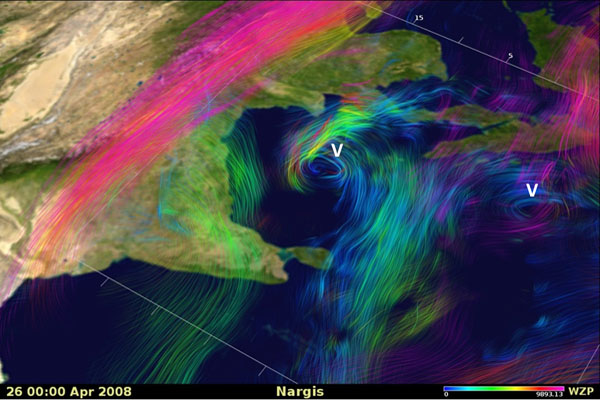
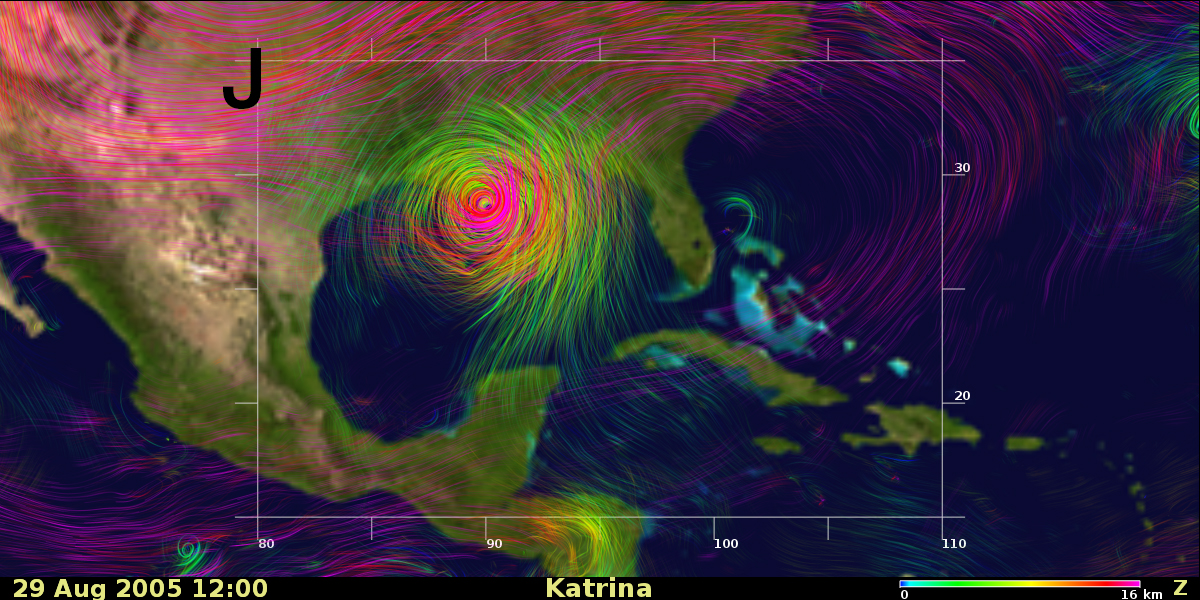
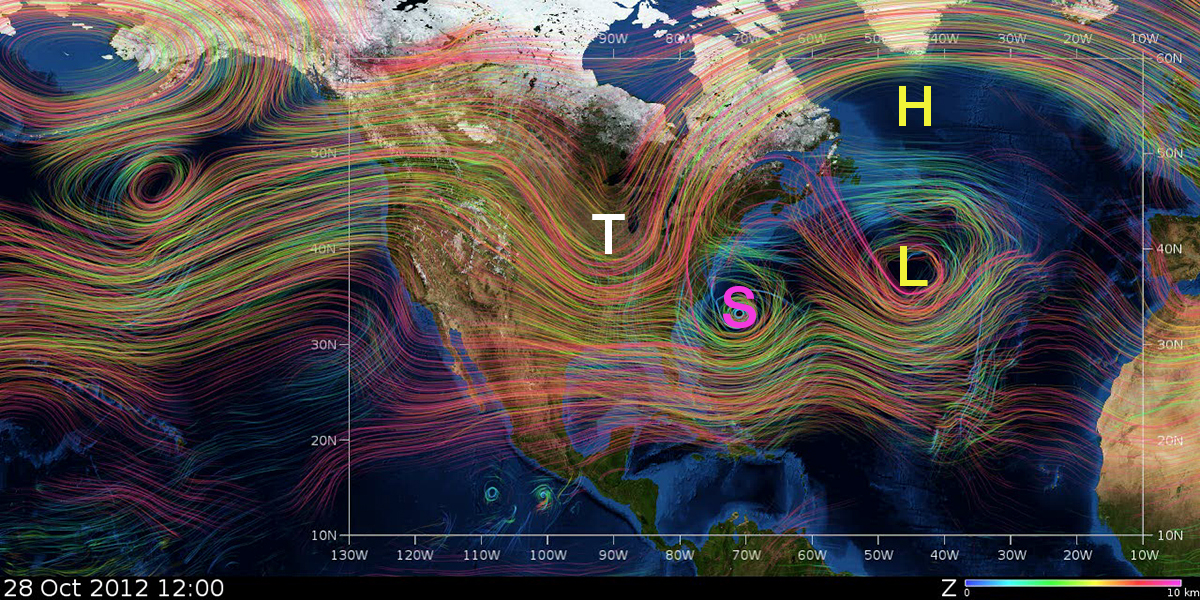
|